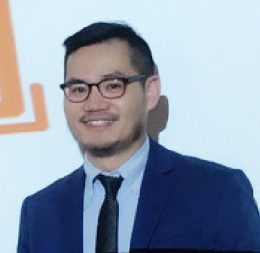
Lingwei Chen, an assistant professor in the Department of Computer Science and Engineering, is exploring data-driven methods that can create cyber-attack detection models.
Wright State University received a $174,943 federal grant for science, technology, engineering, and mathematics research to find ways to build more efficient cyber-attack detection models using machine learning.
Lingwei Chen, Ph.D., an assistant professor in Wright State’s Department of Computer Science and Engineering, said building these cyber-attack detection models generally requires the collection of massive amounts of high-quality labeled attack samples, which is both time-consuming and expensive.
Further, the acquired data sets often do not fully represent the true data distribution. These labeled data scarcity and imbalance issues can change the behaviors of the models and cause them to make incorrect predictions.
Chen said the goal of the research is to explore new data-driven methods to create cyber-attack detection models that can still learn effectively from limited or imbalanced cyber data in a cost-efficient manner.
The models would also be developed as general frameworks that can be used to detect a variety of cyber threats, where any kind of security detection task can be fed in, such as malware, phishing, fraud and intrusion.
“If you don’t have a large pool of labeled data, this presents two different challenges,” said Chen. “The first challenge is that there is limited data to train your model. The second is data scarcity may further introduce a data imbalance issue across a long-standing attack and its newly emerging variants. To address these challenges, this research leverages machine learning to detect cyber-attacks from a more practical perspective and create attack detection models directly based on these problematic attack samples that can mitigate their impacts on the detection performance.”
Chen received his master’s degree in computer science from Beijing Normal University and his Ph.D. in computer science from West Virginia University, where he studied cyber security and machine learning. He was a postdoctoral scholar in the College of Information Sciences and Technology at the Pennsylvania State University.
His research interests include machine learning and security, with an emphasis on developing machine learning solutions to address security-related problems, and investigating trustworthy machine learning to enhance the robustness, privacy and fairness of intelligent systems.