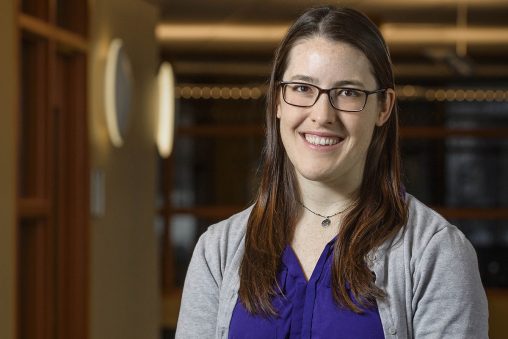
Joy Gockel, assistant professor of mechanical and materials engineering, researches many aspects of additive manufacturing processing.
Two Wright State University researchers have received a $500,000 federal grant to investigate a way to accelerate and improve the manufacturing of thermoelectric materials through additive manufacturing and machine learning.
The U.S. Department of Energy awarded the two-year grant to principal investigator Joy Gockel, assistant professor of mechanical and materials engineering, and Tanvi Banerjee, associate professor of computer science. Gockel’s research includes many aspects of additive manufacturing processing. Banerjee’s research includes multimodal data fusion and machine learning.
Gockel said it usually takes a long time to develop a new material and that trial-and-error approaches are expensive.
“The proposed approach will speed up the development process and allow for the use of additive manufacturing to make high quality thermoelectric material. This method will also be applicable towards the development of different materials in the future,” she said.
The two Wright State researchers are collaborating with Saniya LeBlanc, an associate professor at George Washington University, and Wright State alumnus John Middendorf at ARCTOS Technology Solutions in Beavercreek.
The project, under the Department of Energy’s Office of Energy Efficiency and Renewable Energy’s Advanced Manufacturing Office, is titled Accelerating Additive Manufacturing Process Design for Energy Conversion Materials using In-situ Sensing and Machine Learning.
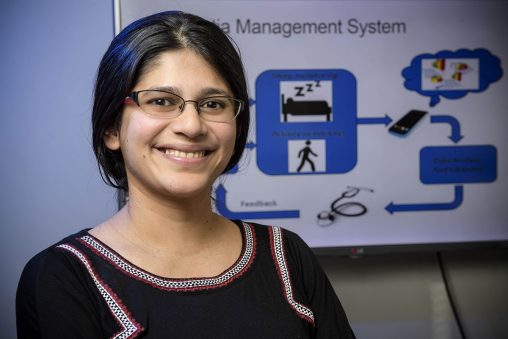
Tanvi Banerjee, associate professor of computer science, researches multimodal data fusion and machine learning.
Additive manufacturing builds 3D objects by adding layer upon layer of material. Machine learning is the use of artificial intelligence that enables systems to automatically learn and improve from experience without being explicitly programmed.
Thermoelectric devices create a voltage when there is a different temperature on each side. So they can be designed to directly convert temperature differences to electric power.
Current thermoelectric device manufacturing processes have critical problems that include assembly of many components, material waste and limited device geometries. The processes, for example, cause more than 50% of the thermoelectric material to be wasted.
One promising candidate as a new manufacturing process is laser powder bed fusion (LPBF) additive manufacturing because it can create multiple device components of desired geometry and complexity in one process with minimal waste. LPBF spreads a thin layer of powdered material across a substrate and the desired geometry on that layer is selectively melted by a laser. The substrate moves down and then another layer of powder is spread, and the process is repeated.
This additive process allows for the fabrication of complex geometric shapes. However, there are many individual pieces of this technology that need to be developed before full device fabrication can be realized.
The project focuses on the manufacturing processing parameters for LPBF of bismuth telluride, a thermoelectric material. The feasibility of using LPBF additive manufacturing for thermoelectric material has been demonstrated by LeBlanc’s research group, but the manufacturing processing parameters need to be optimized.
The large amount of data collected and the many inputs and outputs for successful additive manufacturing processing development make it well suited to machine learning techniques. The project will use sensors developed at ARCTOS and material characterization to enable a machine learning approach.
“There will be a lot of data,” said Gockel. “The biggest challenge will be manipulating the data into a format that is both informative and useable for the machine learning algorithms. The collected data will be used to train and test machine learning models that can be used to optimize the manufacturing process for improved performance.”