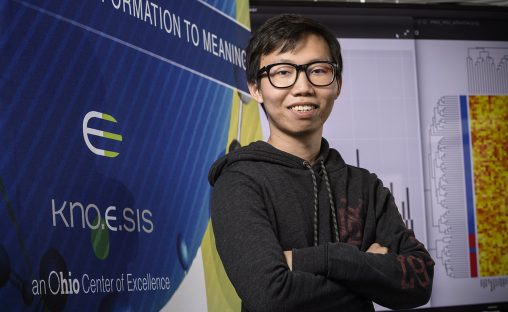
Soon Jye Kho, a computer science Ph.D. student in Wright State’s Kno.e.sis Center, was recognized at the precisionFDA Mislabeling Correction Subchallenge. (Photo by Erin Pence)
Wright State University computer science Ph.D. student Soon Jye Kho was named one of the top performers in an international research challenge by the U.S. Food and Drug Administration designed to detect medical mislabeling through computer analysis.
Kho’s entry was among the three top-performing submissions out of 82 submitted by academic and private researchers from around the world in the precisionFDA Mislabeling Correction Subchallenge.
Researchers taking part in the challenge hailed from Denmark, Korea, Luxembourg as well as the Cleveland Clinic and U.S. universities, including Texas Tech and the University of Michigan.
“I feel excited about it and kind of proud,” said Kho, whose research area is using computer science to analyze medical data. “I’ve been able to utilize what I’ve learned in a practical real-life scenario.”
Kho, who grew up in Malaysia, is a Ph.D. student at Kno.e.sis, Wright State’s Ohio Center of Excellence in Knowledge-Enabled Computing. His adviser is Amit Sheth, the LexisNexis Ohio Eminent Scholar, a professor of computer science and engineering, and the executive director of Kno.e.sis.
“Kho’s win is significant in two ways,” said Sheth. “First, it represents one more win for a Kno.e.sis student in a national or an international level competition. Second, it is an important addition to our growing body of research in precision medicine and personalized digital health, topics of significant importance for Kno.e.sis’ role as an Ohio Center of Excellence in BioHealth Innovation.”
The online data challenge occurred from November to December. Researchers participated remotely. Top performers were announced in February.
FDA challenges are designed to find solutions to real-world problems. The objective of the mislabeling challenge was to encourage development and evaluation of computational algorithms that can accurately detect and correct mislabeled samples.
The accidental swapping of patient tissue samples or genetic data can contribute to invalid conclusions and wrong or ineffective treatments.
“When a sample belongs to a healthy individual but is mislabeled as cancer tissue, the physician might prescribe unnecessary treatment to the individual, which could harm them,” said Kho.
Participants in the challenge were presented with 160 tumor samples, with about 15 percent of them containing labeling errors. They were asked to create computational algorithms to model the relationship between clinical attributes, protein profiles and mRNA profiles using the data, then to apply the model to identify and correct mislabeled samples.
“We employed machine-learning techniques where we train the machine to see the pattern in a cancerous genetic profile and the normal genetic profile,” said Kho. “So if there is mismatch between machine prediction and a patient’s diagnosis, mislabeling is suspected and we could go back to reaffirm the labeling before performing any downstream analysis.”
Computer analysis is becoming a powerful approach to understanding disease and speeding the translation of new discoveries in the labs to patient care.
Kho believes the outcome of the challenge can have a real impact. After earning his Ph.D., he would like to conduct research in academia or the private sector.
“I’m interested in precision medicines and translational research that speeds up findings in the lab to the clinical side,” he said.